资讯 News
目标
Aims
本项目致力于为实现沿海水域浮游生物的长期、连续和高频监测提供创新技术手段,以支持蓝色经济的发展。中科院深圳先进院的工程师团队和澳大利亚联邦科学与工业机构大气与海洋研究所的科学家团队将发挥各自的专业优势,将先进的原位成像技术与人工智能技术相结合,研发下一代基于智慧物联的浮游生物关键类群识别与定量解决方案,为全球气候变化背景下蓝色经济发展所面临的水产养殖监测、有害藻华预警、中型浮游生物动力学、珊瑚礁保育等挑战提供科技支撑,为中澳有关典型海区构建模型提供数据支撑。
This project develops new tools that can achieve long-term, continuous and high-frequency monitoring of marine plankton in coastal waters to support development of the blue economy. By leveraging expertise from both scientists and engineers from the SIAT of CAS and the O&A of CSIRO, and linking cutting-edge camera technology to artificial intelligence for automatically identifying plankton, we will develop next generation solutions for identifying and estimating the abundance of key plankton groups. This work will support the blue economy including aquaculture monitoring (harmful algal blooms and mesoplankton dynamics), coral reef conservation and protection under climate change, and providing data for regional models.
技术 Technology
浮游生物成像仪
Image Plankton Probe (IPP)
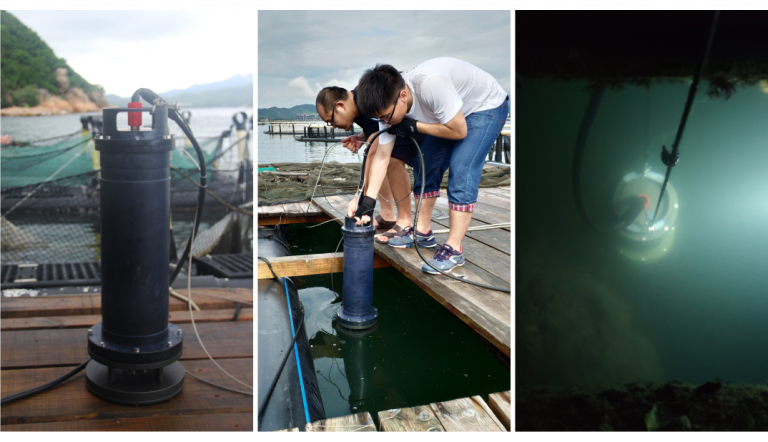
海洋浮游生物观测是海洋环境监测和海洋科学研究的基础。先进院团队已经开发了一种新型的浮标基原位浮游生物成像仪技术。通过结合光学成像、仪器工程、人工智能和物联网技术,原位成像仪可以长时间在水下自动采集活体浮游生物的高质量水下图像,并利用无线网络将图像数据传输至陆地,进而对浮游生物图像识别分类。我们希望这一技术不仅可用于生物多样性和渔业资源调查、赤潮和有害藻华监测、滨海核电站冷源安全、外来物种入侵监测等应用,还可部署于浮标观测网、海底观测网和水下机器人等海洋观测平台,为未来的海洋观测和科学研究提供支持。
Observation of plankton is indispensable for the safety of the marine environment and marine scientific research. We have developed an in situ plankton imager that can work autonomously under a buoy. By combining optical imaging, instrument engineering, artificial intelligence and the internet of things, the instrument can automatically capture high-quality underwater images of live plankton for a long time, transmit image data through 4G cellphone network, and recognize the plankton taxa. We hope the technology can not only be used for surveys of marine biodiversity and fishery resources, monitoring of red-tide and algal blooms, clogging species breakout near coastal nuclear power plant, and alien species invasion, but can also be used for ocean observation platforms such as buoy monitoring networks, seafloor observatory networks and AUVs, serving ocean observation and scientific research in the future.
团队 Team
研究
Research
- Lin Z, Zhan P, Li J, Jun Sasaki, et al. Physical drivers of Noctiluca scintillans (Dinophyceae) blooms outbreak in the northern Taiwan Strait: A numerical study, Harmful Algae, Volume 133,2024.
- Chen T, Li J, Ma W, et al. Deep focus-extended darkfield imaging for in situ observation of marine plankton. Front. Mar. Sci., 2023, 10:1074428.
- Yang Z, Li J, Chen T, et al. Contrastive learning-based image retrieval for automatic recognition of in situ marine plankton images. ICES Journal of Marine Science, 2022, fsac198.
- Guo G, Lin Q, Chen T, et al. Colorization for In situ Marine Plankton Images. ECCV 2022. Lecture Notes in Computer Science, vol 13698. Springer, Cham.
- Li J, Chen T, Yang Z, et al. Development of a Buoy-Borne Underwater Imaging System for In Situ Mesoplankton Monitoring of Coastal Waters. IEEE Journal of Oceanic Engineering, 2021, 47(1): 88-110.
- Yang Z, Chen T, Li J, et al. Focusing Evaluation for In situ Darkfield Imaging of Marine Plankton. OCEANS 2021: San Diego–Porto. IEEE, 2021: 1-8.
- Ma W, Chen T, Zhang Z, et al. Super-resolution for in situ Plankton Images. Proceedings of the IEEE/CVF International Conference on Computer Vision. 2021: 3683-3692.
- Li J, Yang Z, Chen T. DYB-PlanktonNet. IEEE Dataport, 2021.
- Eriksen R S, Davies C H, Bonham P, et al. Australia’s long-term plankton observations: the integrated marine observing system national reference station network. Frontiers in Marine Science, 2019: 161.